Poker-playing AI beats pros using 'intuition,' study finds
The program is designed to play a complex form of poker.
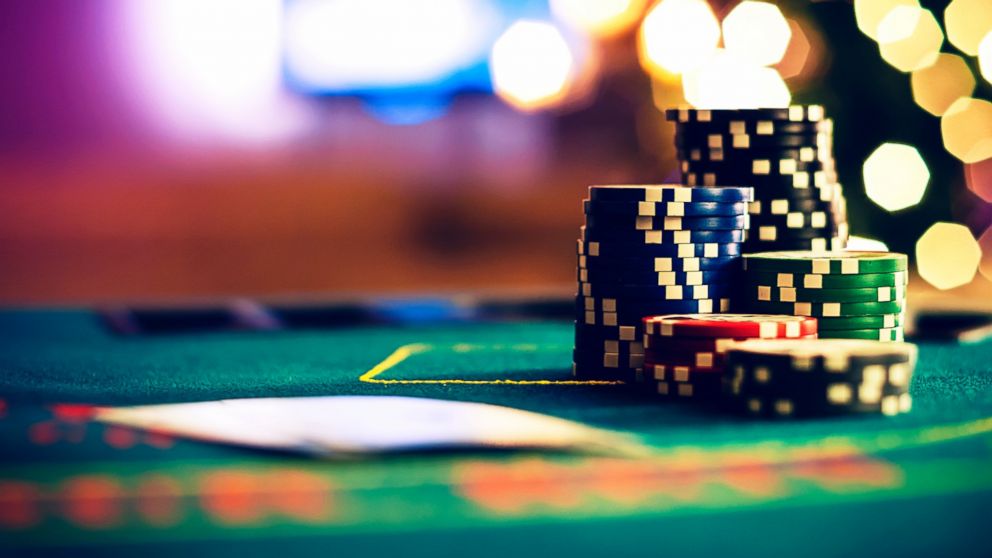
— -- Computer researchers are betting they can take on the house after designing a new artificial intelligence program that has beat professional poker players.
Researchers from University of Alberta, Czech Technical University and Charles University in Prague developed the "DeepStack" program as a way to build artificial intelligence capable of playing a complex kind of poker. Creating an AI program that can win against a human player in a no-limit poker game has long been a goal of researchers due to the complexity of the game.
Michael Bowling, a professor in the Department of Computing Science in the University of Alberta, explained that computers have been able to win at "perfect" games such as chess or Go, in which all the information is available to both players, but that "imperfect" games like poker have been much harder to program for.
"This game [poker] embodies situations where you find yourself not having all the information you need to make a decision," said Bowling. "In real-life situations, it's a rare moment that we have all the information."
There have been other poker-playing AI programs, but they were playing a poker game that included a pot limit, meaning there were limitations on the amount of money could be bet during different stages. As a result, there was less information and risk analysis for the program to compute. In those programs, Bowling explained, the program could look at all potential paths and probabilities for playing different hands prior to playing the game and then simply plug in the information from each hand to win the game.
In this new version of a two-person Texas hold'em poker, there were no limits on betting vastly expanding the amount of information that would need to be processed. Bowling explained without that limitation there were more potential outcomes "than there are atoms in the universe."
"DeepStack gets around that by not pre-computing everything in advance, it will process information at each time," said Bowling.
The programmers were able to create an "intuition" program system for the AI that would focus on looking at each hand in real time and then compute the probability of winning the next few hands, rather than the entire game.
"It only looks a few answers ahead," Bowling explained.
In order for the program to be able to respond in real time, Bowling and his co-researchers were able to create special machinery designed to "learn" complex information. Called a deep neural network, the technology allows the AI to "learn" by looking at past poker games and their outcomes. By simulating poker games over and over, the AI is able to better estimate how to play a hand and figure out a hand's "value."
Bowling explained the program could see via the simulations "how much money would I expect to win if I found myself in this situation."
"If it's positive, it's good for me; if it's negative, it's bad," Bowling said.
The "intuition" could then determine if a hand was more valuable by looking at past simulation results and then be able to better predict a winning move.
To test if their AI could win, the researchers worked with the International Federation of Poker to recruit players willing to play against DeepStack. In four weeks, they had 11 professional poker players each play 3,000 games against DeepStack. They found DeepStack won most of the time against all the players.
"We were ahead by quite a large margin," Bowling said. When they went back to look and see if the program might have just been lucky, they found the program was likely ahead due to skill not luck when pitted against 10 of the 11 participants.
The researchers hope the program will be able to be used for other complicated situations such as "defending strategic resources" or making difficult decisions in medical treatment recommendations.
"With many real-world problems involving information asymmetry, DeepStack also has implications for seeing powerful AI applied more in settings that do not fit the perfect information assumption," the authors said.