How our polling averages work
An explanation of the methodology for 538's current polling averages.
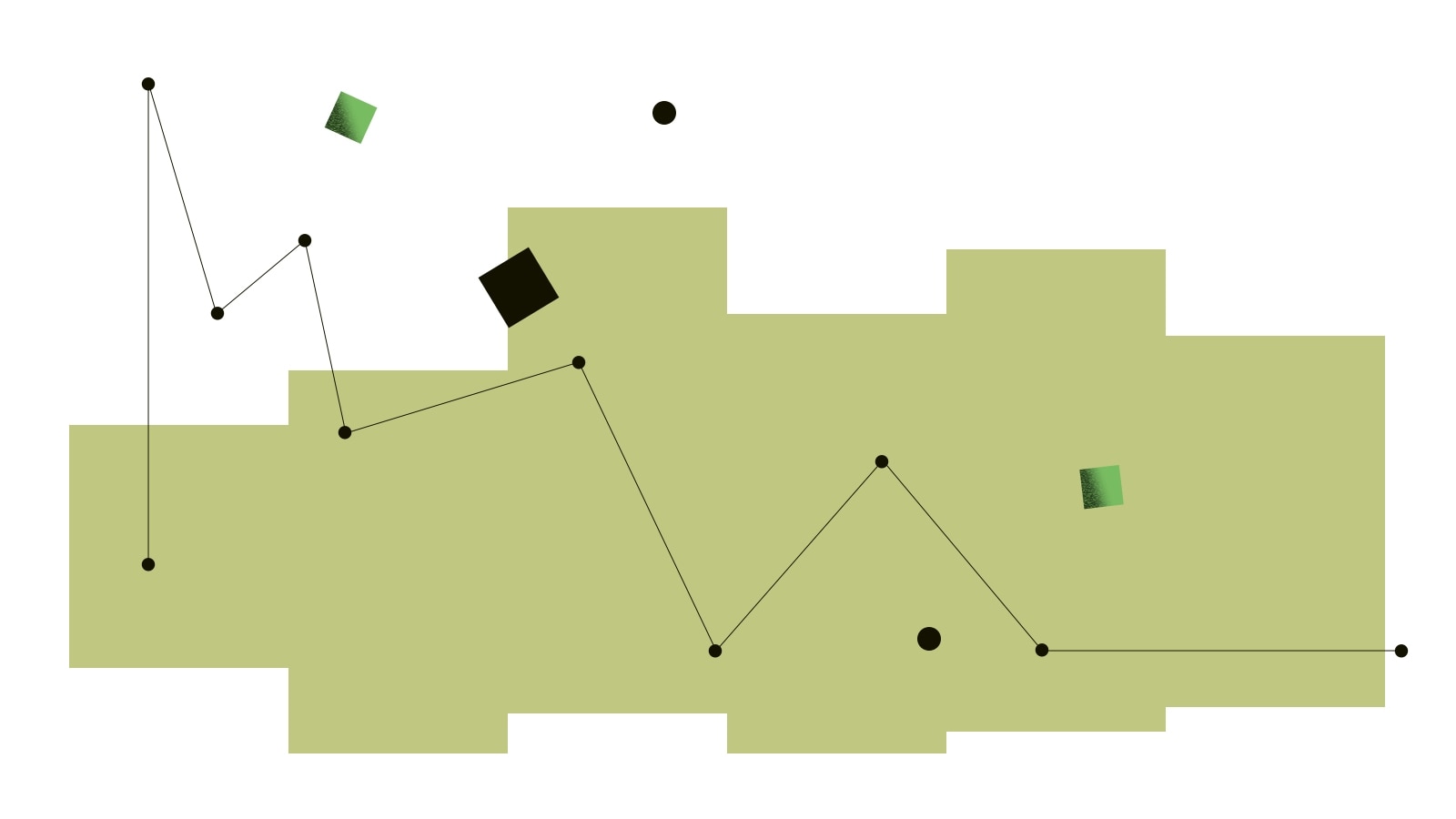
This is an old version of our polling average methodology. For the most up-to-date version, please click here.
**
Almost since its founding, 538 has published comprehensive averages of polls for a wide variety of questions related to U.S. politics. In June 2023, we debuted a new set of models for these averages that aims to improve the accuracy of the underlying models and how the results are visually conveyed to our readers.
The most important differences from our old polling-average model are:
- We now use separate models for each type of polling average. Polling averages need to process information differently in different contexts: For example, presidential approval ratings and horse-race polls can change faster than favorability ratings and generic ballot polls. To account for these variations, we now derive separate sets of parameters to control the aggressiveness of each of our polling averages for presidential and vice-presidential approval, congressional and Supreme Court approval, politician favorability and different types of horse-race averages (presidential elections, presidential primaries, senate and gubernatorial general and primary elections, and the generic congressional ballot), using our historical database of polls.
- House effects can change over time, and have more uncertainty. We don’t want to make unnecessarily large adjustments to a pollster’s house effect as a result of a poll conducted when one candidate happened to be surging, so our house effects are now calculated using the value of the polling average on each day of the time series, rather than the average over the entire time period. Our house effects are also now formally Bayesian, which means the value we end up using in our final average is higher when (a) we have more polls for that pollster and (b) the individual differences from the average for each poll are more narrowly distributed.
- We dynamically adjust our average based on two different aggregation models. We want to avoid scenarios where there aren’t many polls for a while, then a deluge of new data creates a whiplash in the average. To help with that, when we have more data in a recent time period, we rely less on our slow-to-update exponentially weighted moving average and more on our more aggressive polynomial regression trendline. This also has the benefit of giving us averages that are more responsive to quick movement in the data when multiple polls reflect that movement, without reacting too aggressively to any one individual survey showing a big change.
- We calculate uncertainty for every average. Previously, we only displayed uncertainty intervals for our presidential approval average. Now, to visualize the noisiness and estimated error across each of our models, we show uncertainty intervals for all the various types of averages we run.
Here are all the steps we take to calculate our averages:
Which polls we include
538’s philosophy is to collect as many polls as possible for every topic or race we’re actively tracking — so long as they are publicly available and meet our basic criteria for inclusion. After determining that a poll meets our standards, we have to answer a few more questions about it before sending it off to the various computer programs that power our models.
- Which version should we use? If a pollster releases multiple versions of a survey — say, an estimate of President Biden's approval rating among all adults and registered voters — we choose the survey that best matches either the breakdown of polls in our historical database or the preferred target population for that type of poll. In practice, that means if historical polls on a particular topic (for example, presidential approval or favorability ratings) were mostly published among all adults, we will prefer polls of all adults to polls of registered voters and polls of registered voters to polls of likely voters. But for polls of a primary or general election, where we are mainly interested in the subpopulation of Americans who are likely to (or at least able to) vote, we prefer polls of likely voters to polls of registered voters and polls of registered voters to polls of all adults.
- Does this matchup reflect something that could happen in reality? For horse-race polls, we exclude polls that ask people how they would vote in hypothetical matchups if those matchups have already been ruled an impossibility, such as after each party has chosen its nominee or if the matchup doesn’t include an incumbent who’s announced a reelection bid. We also exclude polls that survey head-to-head matchups in races with more than two major candidates or polls that pit members of a ticket against each other (e.g., 2024 Democratic primary polls that include both Biden and Vice President Kamala Harris).
- Does the poll satisfy our polls policy? In addition to excluding polls for all pollsters that don’t meet our standards, individual surveys may also be excluded for other methodological reasons, which we explain in detail on our polls policy page.
- Is this a tracking poll? Some pollsters release results of surveys that may overlap with each other. We account for this potential overlap in these so-called "tracking" polls by running through our database every day and dynamically removing polls that have field dates that overlap with each other until none are overlapping and we have retained the greatest number of polls possible for that series and firm, paying special attention to include the most recent poll.
- Is it an especially large survey? When polls are fed into the model, we decrease the effective sample sizes of large surveys. Leaving these large numbers as they are would give those polls too much weight in our average. As a default, we cap sample sizes at 10,000. Then, for all polls conducted for a given context (say, approval ratings), we use a method called winsorizing to limit extreme values.
- Do we know the sample size? Some pollsters do not report sample sizes with their surveys, especially for polls released a long time ago. While we can usually obtain this number for recent surveys by calling up the firm, we have to make informed guesses for past data. First we assume that a missing sample size is equal to the median sample size of other polls from that same pollster on the same topic (i.e., favorability, approval or horse-race). If there are no other polls conducted by that firm in our database, we use the median sample size of all other polls for that poll type.
How we weight and adjust polls
After all this data is in our database, we compute three weights for each survey that control how much influence it has in our average, based on the following factors.
- Sample size. We weight polls using a function that involves the square root of its sample size. Specifically, we take the square root of a given poll’s sample size and divide it by the square root of the median sample size for all polls of the given poll’s type (i.e., favorability, approval or horse-race). We want to account for the fact that additional interviews have diminishing returns after a certain point. The statistical formula for a poll’s margin of error — a number that pollsters (usually) release that tells us how much their poll could be off due to random sampling error alone — uses a square-root function, so our weighting does, too.
- Multiple polls in a short window. We want to avoid a situation where a single pollster “floods” a race with its data, overwhelming the signal from other pollsters. To do that, we decrease the weight of individual surveys from pollsters that release multiple polls in a short time period. If a pollster releases multiple polls within a 14-day window, those polls together receive the weight of one normal poll. (Our testing suggested 14 days was the optimal window for this calculation.) That means if a pollster releases two polls in two weeks, each would receive a weight of 0.5. If it releases three polls, each would receive a weight of 0.33. To guard against over-punishing very frequent pollsters, we take the square root of this value, and then winsorize it to remove outliers, as our final weight.
- Outliers. Whenever our average sees a new poll, it also calculates how likely it is to be an outlier. First, we calculate the poll’s distance to the nearest survey using a k-nearest-neighbors algorithm. After removing any outliers via winsorizing, we then transform that distance into a probability, representing our confidence that the survey is indeed farther from its “nearest neighbor” survey than we would expect based on the distances observed for other polls and their neighbors.
Second, we calculate a weight for how much influence we give to an outlier poll using a kernel weighting function. Specifically, we calculate the difference between each poll’s result and an iteratively calculated benchmark polling average on the day the pollster finished its fieldwork, and input those values into the kernel weighting function. (We run the aggregation functions described in the next section three separate times: once to establish a baseline, once to adjust that baseline for outliers and once more to detect systematic population and house effects.) The kernel weighting function transforms those values into new numbers that represent the weight we should give each survey in our average. Polls that land further from the average have lower weights. The precise way these values are transformed varies depending on which kernel we pick for the function, but they range from 0 to 1. The kernel weighting function also considers how much the values vary for different types of averages (approval ratings, horse-race averages, etc.), which we calculate directly from the data and then scale by a positive number that gets fed into our model. This gives our model the ability to make the outlier adjustment more or less aggressive depending on the type of data it is aggregating.
We then assign an outlier downweight for each poll based on its distance from the polling average and the confidence we have that it is an outlier. For polls that we are at least 95 percent sure are outliers (using the k-nearest-neighbors algorithm above), we set their weight to the value we computed with the distance-based kernel weighting function. We make sure the downweight is never less than 0.05 for any given outlier poll — equivalent to removing at most 95 percent of its weight in our model. All other polls get a weight of one, which is equivalent to not applying an outlier downweight at all.
Once we have these weights, we calculate a cumulative weight for each poll by multiplying the three component weights together.
The next step is to test and adjust for any factors that could be systematically shifting groups of polls in one direction. We consider three main adjustments here.
- Population adjustments. For each type of survey, we have a preferred sample universe — for example, likely voters for horse-race polling or all adults for presidential approval. Not every poll will use that preferred sample universe, though, so we adjust to minimize variation between different population groups. These adjustments come from a generalized additive model that predicts poll results using variables for the population of each survey, its methodology (whether people were reached online, by phone via a live interviewer, by phone via automated dialer, etc.). The result is an estimate of how much polls from each population category differ from each other when it comes to each of the possible candidates or responses. We use those values to adjust polls from populations we are not interested in to look more like the one we are targeting. We cap the adjustment at ±2 percentage points for any given population.
- House-effect adjustments. Second, we adjust polls for “house effects,” or the tendency for certain polling firms to produce polls that consistently lean one way or another relative to the average poll conducted around the same time. We estimate house effects using a similar formula to the population adjustment explained above, but we compare polls to a baseline polling average without house-effects adjustments. Then, we use tools from Bayesian statistics to make sure the adjustments for a given pollster are not reacting to noise in the data. That’s because what looks like a house effect in an individual poll could just be abnormal amounts of random sampling or other error.
This added step shrinks our model’s initial estimate of a pollster’s house effect back toward 0. We assume that house effects may look large at the beginning of a series but will diminish over time in the absence of other data. Specifically, the regression we run in the adjustment model gives us both an estimated mean and standard deviation for the house effect for each pollster, which we use to update a normal distribution with a mean of 0 and a standard deviation that our model determines using optimization (more details on that below) — usually it’s around 3 points. For national averages, we estimate house effects using only national polls. For state-level averages, we estimate house effects from the polls in that state.
- Trendline adjustments. Finally, for averages of state polls, we apply a trendline adjustment to control for movement in the national political environment between the time the poll was taken and whatever day the aggregation model is run on. This adjustment gives us a better estimate of public opinion in states with sparse polling data. Imagine it’s the 2016 election and you only had polls from Pennsylvania up to Oct. 15, but national polls released up until Election Day. An average of national polls would have shown significant tightening in the race over the last three weeks of the campaign, but an unadjusted average of the Pennsylvania polls would have been stuck at the value of polls taken in mid-October. This simple average would thus have been highly misleading if taken at face value.
How we average polls together
Once we have collected our polls and adjusted them, we can finally calculate a polling average. Our final polling average is actually an average of two different methods for calculating a trend over time.
The first is an exponentially weighted moving average, or EWMA (a popular tool in financial analysis). The EWMA calculates an average for any given day by calculating a weight for each poll based on how old it is, multiplying the poll result by that weight and finally adding the values together. We select the value for a parameter called decay, which determines the rate at which older data points are phased out of the average according to an exponential function. The value of decay is capped at -0.2 to prevent the model from becoming overly aggressive in responding to new data (that’s what the next method is for). It can vary depending on how quickly the average is changing: When the model detects more movement in public opinion in the more recent past, it anticipates slightly more movement on its next update. We also select a value for a parameter called hard_stop, which excludes any surveys conducted after a certain number of days into the past. We make sure this value is between 30 and 60 days, although if there are fewer than 10 polls within the last 30-60 days, the model will consider polls that are further into the past than this window on a day-by-day basis until there are 10 polls.
The second is a trend through points, calculated using a methodology similar to that of the now-defunct Huffington Post Pollster website and the forecasting methodology used by The Economist. We fit this trend using our custom implementation of a kernel-weighted local polynomial regression, which is similar to a lowess regression — a common tool for calculating trends through points on a scatterplot. The trendline and weight on any given poll in this regression depend on two parameters that we also have to set: the bandwidth of the kernel and the degree of the polynomial. (We allow our model to pick between a polynomial degree of either 1 or 2. When there are fewer than five polls in our average, we use a polynomial degree of 0 — which is roughly equivalent to just taking a rolling average of the polls. When there are fewer than 10 polls, we cap the degree at 1.) To guard against overfitting, we take a weighted average of the resulting trendline and a separate trendline calculated with a degree of 0 and a bandwidth equal to the bandwidth of the other trendline times 0.67, giving the better treadline (according to the Akaike information criterion) more weight.
Once the EWMA and polynomial trendlines are calculated, we calculate a mixing parameter to serve as a baseline for how much weight to give each trendline in our final average. This weight depends on the number of polls conducted over the last month. We put more weight on the polynomial regression when there is more data available to estimate it. That has the benefit of giving us less noisy averages when there are fewer polls, because the local polynomial regression detects movement quicker than the EWMA, which is useful when we have news events that move public opinion and coincide with a big dump of new polls.
Next, we average the weights calculated with the mixing parameter with the weights that would have produced the most accurate blended average over the past five days. We determine “most accurate” using a variant of maximum likelihood estimation; each day, we compute two values called EWMA_likelihood and polynominal_likelihood that represent how accurate the EWMA and polynomial trendlines were at predicting the polls over the last five days. The weight we assign to the polynomial is equal to polynominal_likelihood / (polynominal_likelihood + EWMA_likelihood), while the weight on the EWMA is equal to EWMA_likelihood / (polynominal_likelihood + EWMA_likelihood).
Finally, we use a technique called optimization to test the calibration of our model by calculating thousands of different averages for each politician and race in our historical database using different values for each of our seven hyperparameters (parameters that govern the behavior of a model): decay, hard_stop, bandwidth, degree, the mixing parameter, the standard deviation of the house-effects distribution and the scalar for outlier detection. For each type of polling average, our model picks the set of parameters that generate the optimal values for three measures of accuracy:
- The likelihood of our polling average to predict future real poll results. For every time series in our historical database, we calculate an average on every day in the series and then take the log-likelihood of the difference between every poll result and the calculated polling average one day earlier.
- Error autocorrelation of the polls, which captures how well we can predict the differences between polls and the average on a given day based on previous differences between the polls and the average. This ensures that the model strikes the right balance between predicting future poll results and describing past data; a polling average shouldn’t bounce around to match the value of every poll on every day, and neither should it be a straight line on a graph. When autocorrelation is too high, a model is not reacting enough to movement in the underlying data. Too low, and it's reacting too much.
- Autocorrelation of the average. Similar to above, we also check whether our parameters are producing an average that predictably moves up or down over a time period of a few days. Generally speaking, a polling average on any given day should be equally likely to move up or down on the next day it is calculated. But if, for example, we have created an average that usually moves down after it moves up (or vice versa), we can say that trend is responding too aggressively to new polls. Such a trend would have a negative autocorrelation — the average tends to revert to the mean over time. Similarly, if an average is too slow to respond to new data, it will have positive autocorrelation. We want to avoid either kind of autocorrelation!
In 2023, we started calculating these hyperparameters values separately for each type of polling average (that is, presidential approval ratings, favorability ratings, Supreme Court approval ratings, congressional approval ratings, national horse-race presidential primary polls and state-level presidential primary polls). That means that we are always specifying the type of aggregation model that minimizes these three measures of error for that type of polling average. This results in averages that are more reactive to changes in the horse race, which tend to happen as a result of real campaign events, and less reactive to changes in favorability rating polls, which are often due to noise in the data.
And that's basically it! 538’s polling averages can really be thought of as two different models: one that measures any biases resulting from the polls’ underlying data-generating process, and another to aggregate polls after adjusting for those biases.
There is one last feature of note. As with any model we run, polling averages contain uncertainty. There is error in the individual polls, error in our adjustments and error in selecting the hyperparameters that produce the optimal trendlines. Starting in 2023, all our polling averages convey this uncertainty by calculating and displaying the 95th-percentile difference between the polling average on every day and the polls published those days. (Previously, this was only the case for our presidential-approval averages.) This “error band” represents the uncertainty in that average. (Importantly, this measures our uncertainty when it comes to predicting future polls, but it does not measure our uncertainty at predicting future election results. That step comes later, in our forecasting models.)
Finally, while we have tried to squish all of the obvious bugs in our programs, we are always on the lookout for anything we might’ve missed. If you spot something that you think is a bug, drop us a line.
CORRECTION (Oct. 10, 2023, 4:40 p.m.): A previous version of this methodology wrongly stated that the outlier downweight was calculated by multiplying the probability that a poll is an outlier by the weight calculated using the kernel weighting function. Instead, the outlier probability is used only to determine whether to use the weight calculated using the kernel weighting function at all.
In addition, a previous version of our polling averages wrongly did not cap the sample sizes of large surveys at 10,000, as specified in this methodology. The averages have been corrected.
Version History
1.1 | Improved outlier detection, more stable averages, better optimization. | Oct. 9, 2023
1.0 | New favorability, approval and horse-race averages debuted. | June 28, 2023
References
Presidential Approval Tracker | 2024 Presidential Primary Polls | 2024 Presidential Candidate Favorability Trackers